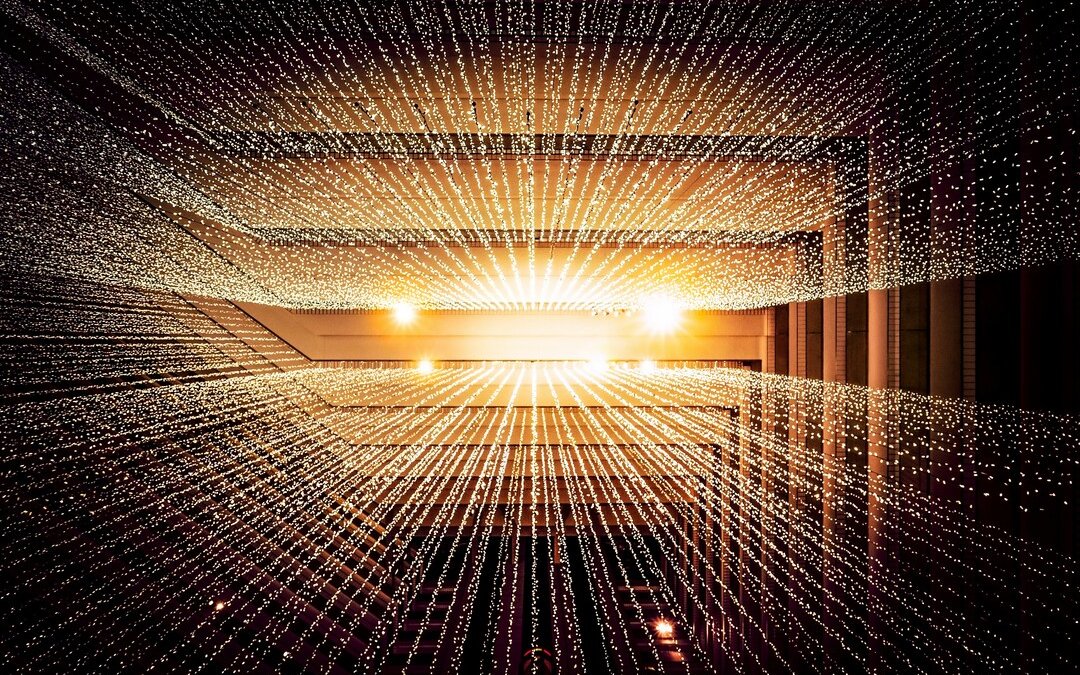
Increasing advancements in building digitization, smart sensor technology, and metering technologies have allowed large amounts of data to be collected and saved for monitoring, analyzing, and controlling building systems. A high-quality data set from the building automation system (BAS) is an essential prerequisite for implementing these technologies. However, the databases obtained from the BAS are usually incomplete due to malfunctioning equipment or sensors, communication issues, power outages, or random interferences that may cause sensors to fail to record data. Further issues may occur when uploading or downloading data to cloud services.
The common characteristics of raw data are:
- Raw data is collected directly from sources and remains unaltered, containing errors, duplicates, and irrelevant information.
- Data often exists in large volumes and comes in diverse forms, making management and analysis challenging without specialized tools.
- The veracity or reliability of raw data can vary, with potential inaccuracies and incomplete information due to uncontrolled collection methods.
- Raw data provides detailed and granular insights, offering deep analysis potential but also posing challenges in data privacy and management.
- While containing valuable insights, raw data requires significant processing and cleaning to uncover meaningful information.
The issue of missing data exists in almost all kinds of data sets, and the extent of the missing data can significantly affect the outcome. For instance, data-driven strategies such as Fault Detection and Diagnosis (FDD) are insufficient in cases where critical functions of the system may have already failed.
Many approaches have been developed for handling missing values, with the simplest approach being to ignore them and perform the analysis based on the available data or through data imputation. Data imputation varies from simple methods such as mean imputation to more robust methods that leverage the relationships among variables within the dataset [I].
Contact Helicon Technologies to manage your data and access your data through a single API.
[i] Pradhan, Ojas; Hälleberg, David et. al. "Lagged-kNN Based Data Imputation Approach for Multi-Stream Building Systems Data" (2022). International High P erformance Buildings Conf erence. Paper 393.